Science Says
Think Smarter, Not Harder: How to Implement Lessons Learned For Business Growth According to Neuroscience
We tend to think of learning as a formal or distinct process, like pursuing a master’s degree, taking a course, reading a book, or listening to a podcast. But learning also happens every day, with every experience, interaction, conversation, execution of a small or big project, and everything in between.
Everything we encounter becomes a kind of input to our minds, but what does the brain do with these inputs? How does it choose what to remember, what to forget, and what to transform into a lifelong memory or core skill?
This new study proposes a theoretical model to capture this kind of experiential learning. The researchers think how we decide what we commit to short-term memory versus what we learn and apply in future situations is quite similar to how neural networks are trained and function. Think of the underlying technology of ChatGPT, Bard, Jasper, and DALL-E other generative AI.
Can we possibly ‘learn better’ from our experiences if we trained like these neural networks? Let’s examine some of the ideas presented in the study.
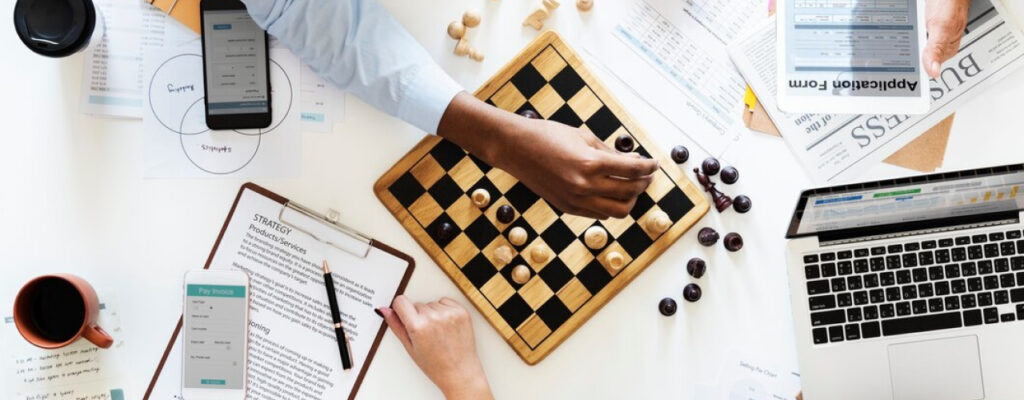
We remember and learn based on predictability and patterns in input or experiences
Do you remember the first few times you tried learning new software on your own by just trying it out, like your project management or bookkeeping tool?
At first, nothing made sense and you had to take screenshots and make detailed notes. After a few tries, you begin to understand the bigger picture of how the tool is structured, where you need to go to do ‘x’, and what happens when you click ‘y’.
This new study proposes that this learning process is similar to neural network training. A neural network is fed a large amount of data and it runs mathematical models to capture the relationship among these data points. Through an iterative process, the neural network adjusts these internal models until they can effectively predict or produce the target or desired output. In the case of us learning a new tool, it’s equivalent to us exploring the interface, clicking around, and figuring out for example that inputs to a particular field and hitting a specific button create a workflow that we track until completion.
This iteration to create a predictive model is what the researchers call ‘consolidation and generalization.’ They believe this is how learning occurs: when our brain sees relationships and patterns in our experiences that are useful for predicting or understanding future similar situations, those inputs, relationships, and patterns are saved in long-term memory.
Learning a tool like software is a very simple example since all the relationships in that system are well-defined, fixed, and predictable. But we also learn from patterns we see in our day-to-day experiences.
You might notice that sending an email at 6:30 PM gets a particular client to open and respond more often than sending it in the morning. Soon you start to notice that other clients of a similar profile (working professionals with families) also seem to open emails sent that time of day. Through repeated exposure to that type of experience and recognizing the pattern and relationships among actions and responses, you come to conclude that it is best to send emails towards the end of the day for a certain subset of your clients.
Action Item: Learning is about finding patterns, relationships, and predictability. But as is often said, “two data points don’t make a trend.” Just like neural networks, we have to go through a sufficient amount of “training data” or experience to find these patterns and predictability.
So don’t give up so quickly on content creation and posting to your social media if it doesn’t seem like you’re figuring out the best way to get engagement and an audience. If a particular skill seems a bit challenging, you may need more practice. When a handful of cold outreach emails don’t get a response, try some more to figure out the effective formula.
BUT sometimes…more training isn’t always better: How noise in data can hinder learning
We probably all have had the experience of obsessing over every detail of an email before sending it out, ‘perfecting’ everything before a product launch, or testing an endless list of software before choosing what social media scheduler to use. We tend to take comfort in believing that more practice and trials lead to better results.
But this study says otherwise. There is a limit to how much practice, experience, and training is optimal for learning. The researchers found that they had to stop feeding training data to the neural network at some point or else additional training data just made its internal model’s predictions less accurate. (What statisticians call ‘overfitting data’.) In the same way, if we go overboard with practice, our brain will keep trying to find patterns and relationships, even when there aren’t any!
For example, you spend so many hours perfecting your social media post and it so happens that you are getting better engagement. Because you have so many repeated experiences of this pattern, you might think there is a causal relationship between hours spent perfecting punctuation, grammar, and formatting and the amount of audience engagement. When in reality, it is your new content and not the new look and formatting that is drawing your audiences in.
This is an example of how the brain might try to force patterns and relationships when there aren’t any, simply because there’s so much data. The more we practice or experience something without proper sense-making and attribution, we tend to pick up more and more little details that we might make a big deal of but just really aren’t material or relevant.
Action Item: To the extent you can, set a limit for training, testing, and practice. Define a clear goal for new skills you are building or a new strategy, and test systematically to determine what you need to do to achieve them.
For example, in trying to figure out the best social media strategy, you can systematically test the time of posting while holding all other factors constant (content, content length, style of graphics, frequency of posting, call to action, etc). Then you can test one other variable while keeping others constant until you can pinpoint the key factor/s that give you the results you need.
Not all experiential learning can be controlled and methodically tested this way. We can, however, be aware of this tendency to see false correlations and patterns. We can also use hindsight and backward-looking assessments to test the validity of some generalizations we hold on to (more on these in the next sections).
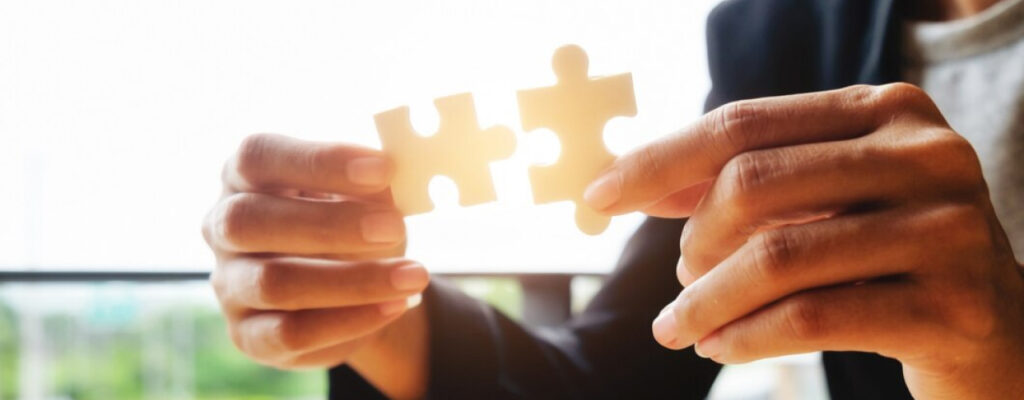
Biases and mental shortcuts can impact memory consolidation and learning
Neural networks can quickly test how accurate their internal models are in predicting target outcomes based on a validation process built into their training. But in real life, we don’t have the benefit of conducting quick validations like these on our beliefs and generalizations. For most of our day-to-day experiential learning, we have no way of knowing beforehand how ‘predictable’ things are, how much training is optimal, or how much ‘noise in the data’ there is we need to dismiss.
So our brain resorts to mental shortcuts called heuristics to process our experiences. For example, you have a Facebook follower who is always complaining about the timing of your free live training sessions. Because of that experience of getting frequent complaints, even if it’s just coming from one person, you conclude that you need to make your sessions earlier.
This is an example of an ‘availability’ heuristic. We rely on information that’s readily available to us to make judgments or conclusions. Sometimes we judge people based on a ‘stereotype’ – because they fit a particular profile, we assume they belong to that category. This is called the ‘representativeness’ heuristic, like when we meet someone at a networking event who looks very young, and we immediately assume they are inexperienced and probably just starting in business.
A third type of mental shortcut is ‘anchoring,’ where you base estimates on the first piece of information or base figure without sufficient research. For example, your potential client says they only expected to pay “x” dollars for your service, and you immediately reconsider if your prices are too high.
Action Item: Quite the opposite of the prior section where we can talk about how trying to accommodate too much in your learning process can lead you astray, jumping to conclusions using shortcuts can also be counterproductive.
Awareness of these biases and heuristics is the first step to mitigating potentially costly mistakes or missed opportunities. Create a data-driven decision-making framework to help come up with a more objective point of view. Give yourself more time to formulate decisions, so aren’t forced to rely on potentially flawed mental shortcuts. Be open to other perspectives and input from those who don’t think like you. Lastly, reviewing past decisions and the outcomes can also guide you to a fairer assessment of previous choices and conclusions (more in the next section).
Assessment and review are key to learning
Periodic evaluations may be the most effective way of checking our biases and the soundness of assumptions and generalizations on which we base our decisions. This would be like the manual version of the iterative error minimization process neural networks use to build their internal computational models. Neural networks refine their formulas until they can predict and produce the expected output in validation data sets as closely as possible.
For us humans, we need to test as well that the patterns, predictions, and relationships we have learned do provide the results we expect. Do our generalizations and beliefs truly have predictive power and are they useful for future situations?
If in your first year of business, you built a healthy pipeline of clients purely by relying on word-of-mouth marketing, you might mistakenly believe that by continuing the good work and maintaining friendly relationships with clients, this will hold for the next year as well. If you have recently hired a VA and have been closely reviewing all the external communication they send out, you might assume you need to review ALL the communication moving forward because the system has worked well so far.
But if we don’t periodically review the usefulness of generalizations such as these, we could be wasting time, resources, and opportunities going down one path when it isn’t doing any good. Periodic reviews or assessments could either confirm, invalidate, or raise questions about assumptions made.
In our earlier examples, you would want to get the figures on whether email open and response rates have indeed improved versus before you changed the time you sent them out. After implementing a change to your social media strategy based on what you determined were the key drivers, you should assess the data on engagement before and after you implemented the changes.
Action Item: Schedule a regular evaluation of assumptions regarding processes or strategies. Are the outcomes as you had hoped, or maybe there is an element of what you are implementing that does not drive the desired outcome?
And it is not just about whether you could have overfit the data, assumed correlation or causation where there wasn’t any or you just didn’t base on enough data; a dynamic environment can change patterns and relationships which we could miss if we don’t pay attention and continuously factor in new experiences and input.
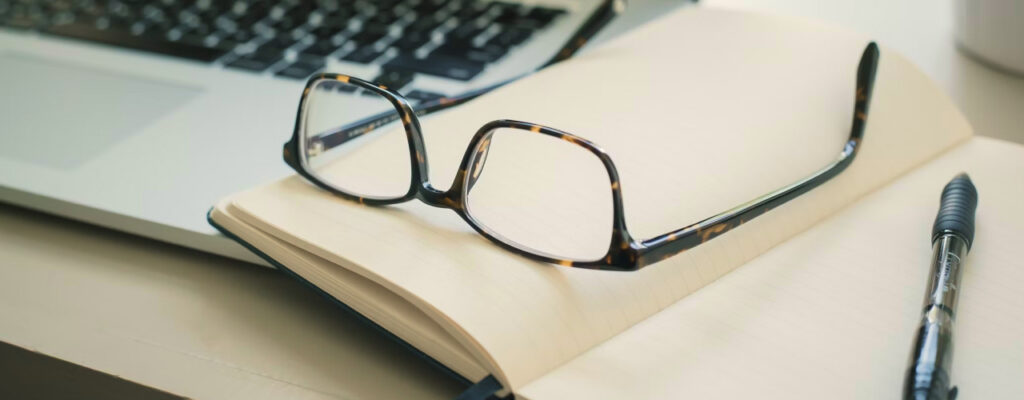
Learning is continuous, adaptive, and flexible
New learning is driven not just by pattern detection and validation – new input, new experiences, and new relationships between existing and new knowledge require that the brain be adaptable and continuously adjust. This study suggests that we will retain and believe what is predictable and useful for future situations, so if we encounter otherwise, the learning process restarts.
Think of how ChatGPT was first released with the caveat that it was trained on data up to September 2021. It could not factor in any events, scientific discoveries, or new pieces of writing that came after that date which could impact its computational models.
Our experiential learning is cumulative and flexible like this as well. Our generalizations can evolve as we are exposed to new information, experiences, and input.
Action Item: Continuous learning is vital, whether formal study or new experiences! This way we can be more in tune with current trends and events, revise previous generalizations, and be more responsive in a dynamic environment.
Wrap Up
Neural networks learn in controlled environments with a mathematically rigorous training process. While experiential learning is much more complex and confounded by a lot of unpredictable ‘noise,’ we can learn from neural networks to apply a certain discipline and structure to how we learn from experience.
There needs to be some volume in training, but just the right amount, and need to be aware of biases and faulty heuristics. Periodic assessment to determine if our learning is accurate and useful as expected will ultimately help us learn better, especially in a fast-changing environment with vast amounts of new input to process every day.
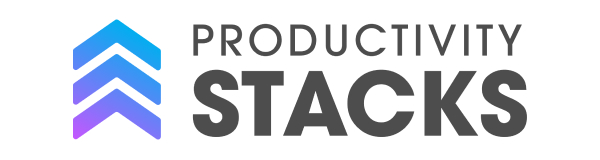